This is the fifth episode in our series on Data Fluency. You can also watch the first episode, second episode, third episode, or fourth episode.
Let’s show you what good data looks like, and what we went through to get it. We wanted to see what happens on the world’s most productive teams. Going into it, we knew that it is possible to measure performance on teams – you can reliably measure it and you do get variation, because some teams perform much better than other teams, and some teams perform much lower. We wanted to know why, and if you could use questions to predict performance.
In essence, we wanted to learn what conditions exist on the most productive teams. It would tell us a lot about employee engagement and leader effectiveness – but the question remained, how can you measure the feelings that the high-performing teams had, but that the low-performing teams didn’t?
Here’s how we started: We asked question, after question, after question to the high-performers and the low-performers. These had to be questions about the individual’s own intentions and experiences (to avoid the Idiosyncratic Rater Effect), so none of them asked the individual to rate someone else on anything.
Secondly, all of the questions had to use extreme language to create variation. Words like “every day” or “great confidence” or “surrounded” or “always.” Using these extreme words meant that we got variation in our answers, with the high-performers choosing “Agree” or “Strongly Agree” across the board, and the low-performers choosing “Disagree” or “Strongly Disagree.”
Lastly, we needed these questions to predict positive outcomes in the future. If someone answered “Agree” or “Strongly Agree” to a question, they needed to have higher performance or retention down the road.
When we did that, we ended up with these 8 questions:
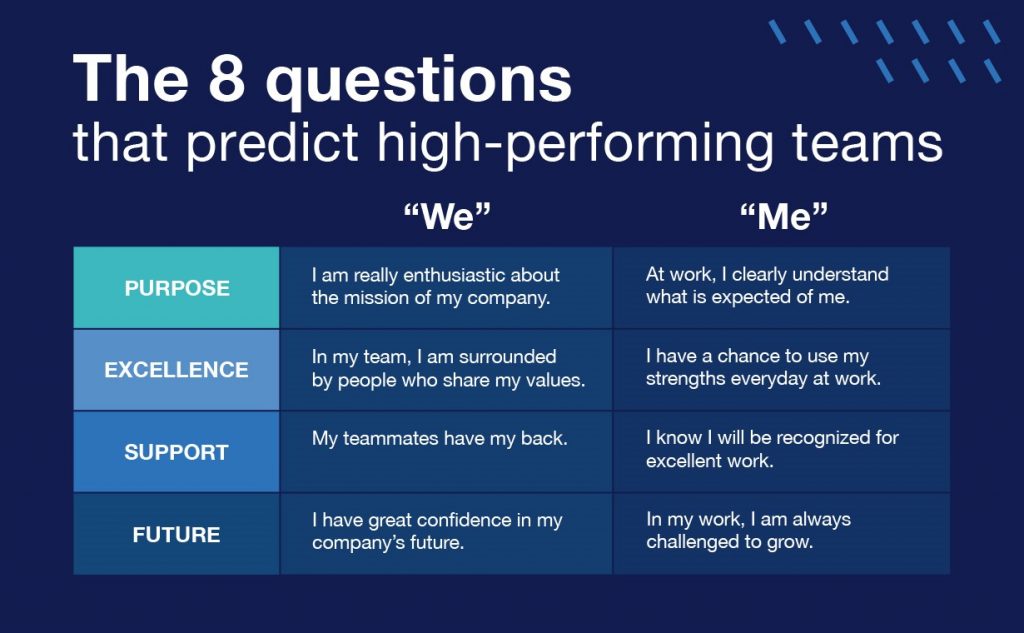
Every question involves the individual rating his or her own experience and intentions. Every question produces range. And every one of them exists in the survey because it predicts future performance.
You can predict performance with these 8 questions.
Tweet thisThat’s what good data looks like, and that’s what you need to go through to get it. It will take about ten years to have confidence in the data, but if your company is making talent decisions – how to pay you, who to fire, when to promote – having data that actually validly predicts future performance is more than helpful – it’s necessary.
These eight questions are used in the Engagement Pulse, a part of StandOut – the technology solution to Talent Activation. To learn more, go to www.TMBC.com or click here.
Note: The views expressed on this blog are those of the blog author(s), and not necessarily those of ADP. This blog does not provide legal, financial, accounting, or tax advice. The content on this blog is “as is” and carries no warranties. ADP does not warrant or guarantee the accuracy, reliability, and completeness of the content on this blog.
ADP, the ADP logo and the ADP Research Institute are trademarks of ADP, Inc. All other marks are the property of their respective owners. Copyright © 2020 ADP, Inc.